In an era where data is as valuable as currency, the importance of data quality and ownership cannot be overstated. Organizations across the globe invest heavily in data-driven strategies to enhance their operational efficiencies, customer experiences, and decision-making processes. However, without robust statistical methods to assess the quality and ownership of this data, these strategies can quickly become liabilities rather than assets. This post will explore the critical role of statistical methods in ensuring the integrity and legal standing of data, focusing on key areas such as data annotation assessment, data quality assessment, data literacy assessment, and data-driven assessment.
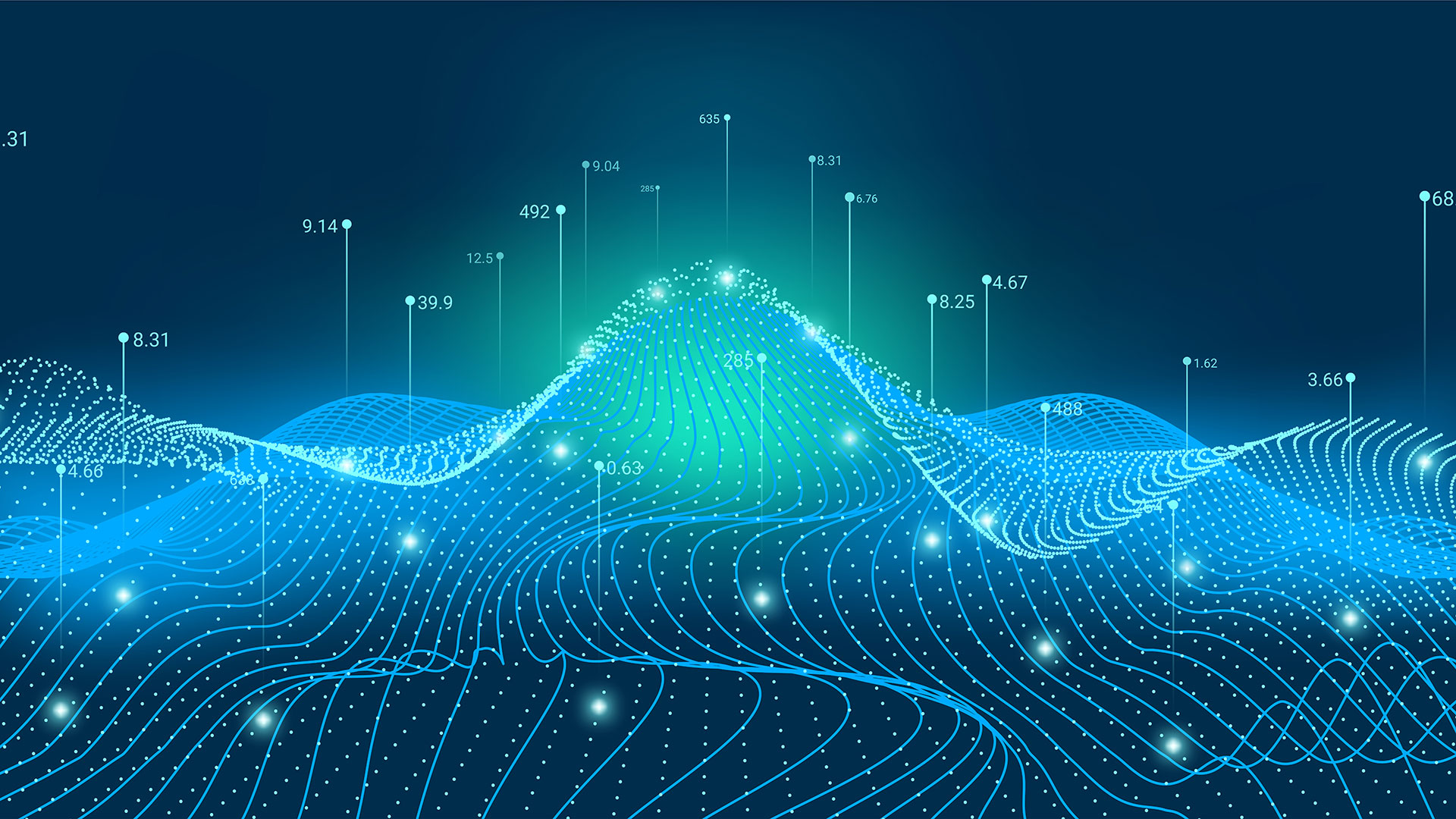
The Foundation of Data Quality: Understanding the Basics
At the heart of any discussion about data quality is the need for a solid understanding of what constitutes ‘good’ data. Good data must be accurate, complete, relevant, and timely. To ensure these qualities, data quality assessment employs a variety of statistical techniques. One fundamental tool is the pattern number calculator, a statistical method that helps identify recurring patterns and anomalies in data sets. By analyzing these patterns, organizations can pinpoint inaccuracies or inconsistencies that may indicate deeper issues with the data collection or processing systems.
Additionally, data annotation assessment plays a crucial role in improving data quality. It involves examining the metadata associated with data sets to ensure that the annotations accurately represent the underlying data. This assessment is particularly important in fields like machine learning and AI, where annotated datasets train models. By applying statistical tests to assess the consistency and reliability of these annotations, data scientists can significantly enhance the accuracy of their models.
Enhancing Data Literacy: A Key to Better Assessment
Data literacy assessment is another critical aspect of maintaining high data quality. This process evaluates the ability of individuals within an organization to understand and use data effectively. Statistical methods used in this assessment include evaluating the outcomes of data-driven decisions and the frequency and types of errors in data interpretation by staff. By identifying gaps in data literacy, organizations can tailor training programs to improve overall competence in handling data, leading to more accurate data-driven assessments.
A data-driven assessment is integral to this process, utilizing data to evaluate its own integrity. This involves statistical analyses such as time-series analysis for trend identification, regression analysis to determine causal relationships, and hypothesis testing to validate data quality assumptions. These techniques allow organizations to continuously monitor and enhance the quality of their data.
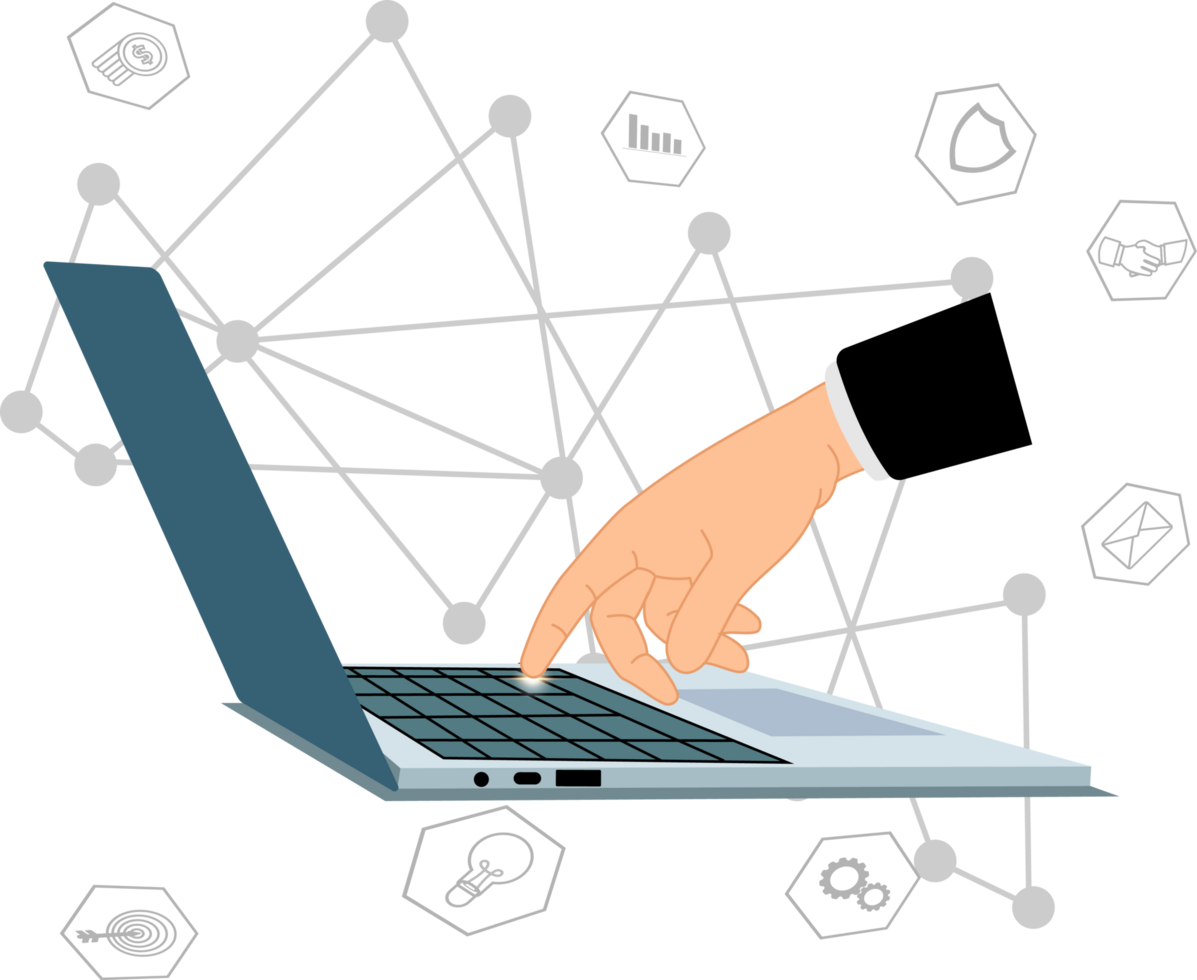
Ownership and Legal Considerations in Data Management
Beyond quality, data ownership is a critical aspect that must be assessed to ensure compliance with legal standards and to maintain the integrity of data management practices. Statistical methods are vital in this arena as well, particularly in identifying and verifying the origins and lineage of data. For instance, cluster analysis can be used to distinguish data sets based on their source or characteristics, which is essential in complex environments where data may come from multiple sources.
Data ownership also involves ensuring that data usage complies with privacy laws and contractual agreements. Statistical audits can be conducted to review how data is accessed, shared, and stored, ensuring that these actions are in line with established protocols and legal requirements. By regularly assessing data ownership through these methods, organizations can mitigate the risk of legal challenges and penalties.
Advanced Techniques for Comprehensive Data Assessments
In today’s data-driven environment, organizations are constantly seeking advanced techniques to ensure their data assessments are thorough and yield actionable insights. These techniques not only focus on enhancing data quality and accuracy but also encompass complex aspects like data ownership and usage compliance. Advanced statistical methods and emerging technologies play a crucial role in this sophisticated landscape, enabling deeper analysis and better decision-making capabilities.
Machine Learning and Predictive Analytics
Machine learning (ML) offers powerful tools for predictive analytics, which can be crucial in preemptive data quality and ownership assessments. By using historical data, ML models can learn to identify patterns that typically lead to data quality issues, such as incomplete datasets or anomalies that could suggest breaches of data integrity. Predictive models can be deployed to flag these issues before they affect the analysis or decision-making processes. For instance, anomaly detection algorithms can automatically scan large datasets for outliers that deviate from expected patterns, offering a proactive approach to maintaining data quality.
Natural Language Processing for Data Annotation Assessment
Natural Language Processing (NLP) technologies enhance the data annotation assessment by automating the extraction and classification of information from textual data. This is particularly useful in managing large volumes of unstructured data, such as customer feedback or social media posts. NLP can be used to tag data automatically, categorize it according to predefined parameters, and even assess the sentiment behind the text. These capabilities make NLP an indispensable tool for refining data quality and ensuring annotations accurately reflect the dataset’s content and context.
Network Analysis for Tracing Data Ownership
Network analysis is a sophisticated method used to map and understand the relationships between different data sources and their respective datasets. This technique is particularly valuable in environments where data ownership is complex and intertwined with various elements. By visualizing these connections, organizations can track data provenance, understand ownership structures, and ensure compliance with data governance policies. Additionally, network analysis can identify central nodes and key actors within data ecosystems, highlighting potential vulnerabilities or control points that could impact data integrity and ownership.
Time Series Forecasting for Continual Assessment
Time series analysis offers a dynamic approach to monitoring data quality and ownership over time. This method analyzes data points collected or recorded at successive time intervals to forecast future values based on previously observed patterns. In the context of data-driven assessments, time series forecasting can help predict trends in data quality, detect seasonal effects, or identify cyclic errors in data collection and processing systems. This ongoing assessment ensures that data remains relevant and reliable for decision-making processes.
Integration of Blockchain for Immutable Data Trails
Blockchain technology is increasingly being explored for its potential to enhance data ownership assessments by creating immutable records of data transactions. Each block in a blockchain contains a timestamp and transaction data, making it an excellent tool for maintaining secure and transparent audit trails. This technology can prove pivotal in scenarios where data custody and historical integrity are critical, such as in financial services, healthcare, and legal sectors. By using blockchain, organizations can ensure that every piece of data is accounted for and traceable back to its origin, significantly reducing the risks associated with data tampering and loss of ownership in the digital commons.
The Way Forward with Data Assessment
The field of data quality and ownership is evolving rapidly, driven by advancements in statistical methods and an increasing reliance on data-driven decision-making. By incorporating comprehensive data quality assessment, data literacy assessment, and sophisticated data-driven assessment strategies, organizations can not only enhance the accuracy and reliability of their data but also ensure compliance with regulatory standards.
In conclusion, as we advance further into a data-centric world, the importance of robust statistical methods in assessing data quality and ownership will only grow. Organizations that invest in these methodologies will be better equipped to harness the true power of their data, driving innovation and maintaining a competitive edge in their respective industries.
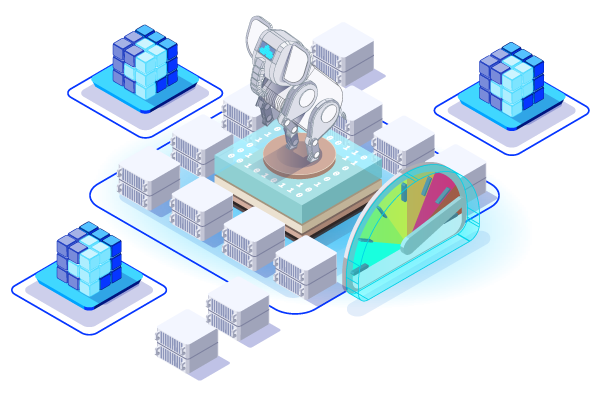